Although the popularity of Big Data is increasing day-by-day, the concept stems from a very simple theory. Businesses mostly rely on transactional data, stored in relational databases, for decision making. However, there’s also a treasure-trove of unstructured, non-transactional data available to them in the form of emails, blogs, photographs, sensors, etc. that can be harnessed for gaining useful insights. Today, since storing and computing data has become more affordable, organisations can utilize this non-traditional yet potentially valuable information for analysis and business intelligence. And this is precisely what Big Data helps companies do – manage volume, velocity and variety of data.
Since Big Data helps businesses organise and analyse diverse data types and capitalise on hidden relationships, it is imperative for them to manage their enterprise big data properly. But not only is moving and transforming it difficult, integrating it with organisational data flows, while also maintaining quality, is also a daunting task. So organisations need to build an efficient Big Data platform based on their needs, the infrastructural requirements for which span:
- Acquisition – Since Big Data taps data streams with high velocity, volume and variety, it entails a major change in the existing infrastructure. The new one should be able to deliver low and predictable latency in capturing data and executing queries; handle high transaction volumes in distributed environments; and support dynamic and flexible data structures. In most cases, NoSQL databases makes for a good choice, as they capture data without classifying or parsing it. They are also highly scalable and better suited for complicated data structures.
- Organisation – High volumes of data need to be organised at their initial destination to save time and money. Thus, the Big Data infrastructures need to be able to process and modify data in its original location; support extremely high throughput in order to deal with large data processing stages; and handle a wide array of data formats, ranging from structured to unstructured. Hadoop allows large volumes of data to be organised as well as processed in their original storage clusters, which makes it increasing popular in the Big Data landscape.
- Analysis – The infrastructure needed to analyse Big Data should be able to support complex analytics, like data mining and statistical analysis, on a wide range of data formats stored across varied systems; scale to high volumes of data; ensure faster response times based on behavioural changes; and automate decisions depending upon analytical models. In addition, it must be able to analyse Big Data in tandem with traditional enterprise data.
Today, the Big Data landscape is dominated by operational and analytical technologies, both of which have evolved differently to address varied demands. While the former focuses on capturing and storing real-time, interactive information, the latter is for complex, retrospective analysis. Being complementary, both of these are often deployed together. Thus, companies can utilize operational workloads to harness cloud computing architectures and scale quickly and inexpensively, and use analytical workloads to tackle sophisticated data sets and scale beyond resources made available by a single server.
Businesses must, therefore, evaluate their Big Data needs before planning the transition to ensure a seamless integration. They should focus on devising a strategy for evolving business architecture to incorporate Big Data with a view to increase business value.
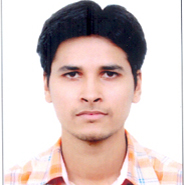
Zubair is a Senior Technical Specialist – Big Data Practice at Happiest Minds. He has 8 years of experience in Big Data (Telecom), Network Acceleration products, Router, ASN Gateway and Networking related projects. He also has extensive experience in Hadoop, Map Reduce, HBase, Python and Shell Scripting and C programming.