Introduction
Biomarkers are defined as disease-associated molecules, mostly genes, proteins, and metabolites, that can be used for the indication of diagnosis, prognosis, and therapeutic responses of diseases. Drug targets are also biomolecules (DNA, RNA and proteins), associated with a specific disease(s) and can be targeted by a drug molecule to produce a therapeutic outcome. The identification of these disease-associated biomolecules is the initial and fundamental step of the drug discovery process. Next-generation sequencing (NGS), a specialized technique, generates high-throughput sequencing data to determine the order of nucleotides in the genomes (DNA/RNA, amino acids in proteins). Here, we are going to discuss the application of different NGS omics technologies (Genomics, Epigenomics, Transcriptomics, etc.) in the discovery of disease biomarkers and drug targets.
Genomics
Genomics technologies (DNAseq) have revolutionized disease biomarker identification by enabling comprehensive analysis of genetic alterations associated with disease onset, progression, and treatment response. Whole-genome sequencing (WGS) and Whole exome sequencing (WES) were applied to detect mutations (nucleotide substitutions, small insertions, deletions, copy number variations, etc.) of several cancer-associated genes, like TP53, PTEN, KRAS, BRCA1/2, assessing the risk of tumorigenesis.
High-throughput genomic data have been used for Genome-wide association studies (GWAS) to capture genetic variants associated with disease risk. Such genomic studies identified genes and ncRNAs that led to new target identification. WES has revealed recurrent mutations associated with drug resistance. Targeted DNA sequencing assessed Tumour mutational burden (TMB) to identify the potential target regions. Single-cell genomics discovered novel tumour antigens as ideal drug targets for monoclonal antibodies in cancer. Single-cell targeted DNA sequencing identified FLT3 resistance of RAS/MAPK mutated cells in acute myeloid leukaemia. Pharmacogenomics focuses on the impact of genetic mutations of individual patients on drug response, providing information on drug efficacy and drug toxicity.
Epigenomics
Epigenetics (DNA methylation, histone modification, microRNA) is associated with the regulation of the transcriptional process without altering the DNA sequence itself. Chromatin immunoprecipitation sequencing (ChIP-seq), Whole genome Bisulfite sequencing (WGBS) and Assay for Transposase-Accessible Chromatin with sequencing (ATAC-seq) now enable systematic analyses of the epigenome, revealing aberrant methylation patterns or miRNA dysregulation that serve as potential biomarkers for early detection, prognosis, and therapeutics. DNA methylation-based studies revealed HOXA9 and HIC1 as diagnostic serum biomarkers for early detection of ovarian cancer. Studies on Histone methylation modification explored specific methylation patterns (H3K9me3, H3K27me3, H3K36me3, etc.), serving as significant markers for several cancers (gastric, liver, pancreatic, and colon cancers).
DNA methylation studies have identified potential therapeutic targets. For example, tumour suppressor genes are silenced in cancer cells by PRC (Polycomb repressive complex) reprogramming (EZH2 catalyses the methylation of H3K27). Polycomb-mediated repression demonstrated a putative target option by the inhibitors of PRC2. The miRNAseq data suggested specific microRNAs (e.g., miR-34) act as tumour suppressors, showing the ability to regulate the expression of multiple oncogenes.
 Transcriptomics
Whole genome transcriptome profiling gives a holistic understanding of the expression alteration of genes under different conditions. RNAseq-based studies have identified IDH as a good prognostic marker for glioma, and COX2 and HER2 as potential diagnostic markers for Colorectal cancer (CRC). Single-cell RNAseq (scRNAseq) has facilitated the discovery of more precise prognostic markers in CRC. Single-cell data analysis from tumour and juxta-tumour regions led to the identification of epithelial cell groups with intrinsic consensus molecular subtypes (CMSs – iCMS2 and iCMS3) and thus facilitated the marker-based disease subtyping.
Bulk RNAseq has been applied to in vitro cell lines and tissues to fetch the gene expression profiling of putative drug targets. This evaluates the biological functions of those target genes under normal, diseased and drug-induced conditions. Single-cell RNAseq (scRNAseq) data analysis enabled the discovery of cell-type-specific targets in cancer (e.g., S100A4 as a novel immunotherapy target in glioblastoma). Spatial transcriptomics data analysis captures the histological context of cells and cross-talk between different cell types in specific tissue structures (e.g., tumour microenvironments).
 Proteomics
Proteomics technologies can monitor, quantify, and characterize proteome (protein content at a given point of time or space) explaining the structural and functional aspects of proteins. Mass spectrometry-based analyses over the last decade have identified differentially expressed proteins across various sources, uncovering potential biomarkers and therapeutic targets. Despite challenges such as small sample sizes, the findings underscore the potential of proteomics to elucidate disease mechanisms and accelerate biomarker validation in larger cohorts. Proteomics profiling has been used to stratify patients with different autoimmune diseases (e.g., rheumatoid arthritis, systemic lupus erythematosus, etc.). Researchers developed a panel of protein markers where SPP24 and α-1 microglobulin were reported to differentiate Inflammatory bowel disease (IBD) from healthy individuals.
As proteins are the main target molecule of most drugs, Proteomics approaches play a crucial role in drug target discovery. Mass spectrometry (MS) based approaches facilitated the study of selectivity and specificity of the putative drug targets and thus elucidated the mechanism of action (MoA). Functional proteomics explains the post-translational phenomena and the protein-protein interaction landscape under various diseased conditions. Chemical proteomics provides a direct approach to assess novel enzymes in physiological conditions and accelerate the target discovery process. Structural proteomics (ligand-based / docking based drug-target interaction) helps to predict putative drug-targets minimizing the cost, time, and clinical risks.
 Metabolomics
Metabolomics data comprises a set of small molecule metabolites derived from metabolism of living organisms. Unlike other omics, metabolomics directly reflects the dynamic interplay between physiological, environmental, and lifestyle factors, making it particularly suited for identifying disease-specific metabolic alterations. In kidney disease, metabolomic studies have identified urinary peptide signatures that complement traditional markers like albumin, implicated the microbiome in uremic states, and linked kidney bioenergetics with acute kidney injury outcomes. Liquid chromatography-mass spectrometry (LC-MS), Capillary Electrophoresis (CE-MS) and Nuclear magnetic resonance (NMR) based metabolomics studies (CSF and plasma samples) established metabolites as biomarkers of Alzheimer’s disease (Acylcarnitines, Arginine, Aspartate, Histidine etc.).
LC-MS-based studies assessed the toxicity and side effects of drug-targets (off-targets of a drug). Several analyses aimed to find metabolites whose levels correlate with disease aggressiveness and further assessed the enzymes producing these metabolites as potential therapeutic targets (e.g., glycine-N-methyltransferase, produces Sarcosine, identified as a potential therapeutic target of Prostate cancer).
Multi-Omics
With the advent of omics technologies, researchers identified the higher complexity of biological systems. To deal with such complexity, the latest studies started integrating data from more than one omics technology (called multi-omics). There is an increasing number of outcomes of multi-omics-based biomarkers for various diseases like, diffuse large B-cell lymphoma, pancreatic cancer, cardiovascular diseases, obesity, diabetes, Alzheimer’s disease, etc. A recent study has integrated genomics, transcriptomics, proteomics and metabolomics and established blood-based biomarkers for Parkinson’s disease. However, multi-omics approaches also face some challenges, such as data integration, standardization, interpretation, reproducibility, and validation. Therefore, more research studies are required to improve the methods which can integrate multi-omics data robustly. More open-source tools and high computing platforms need to be developed to make omics/multi-omics analyses easier for researchers.
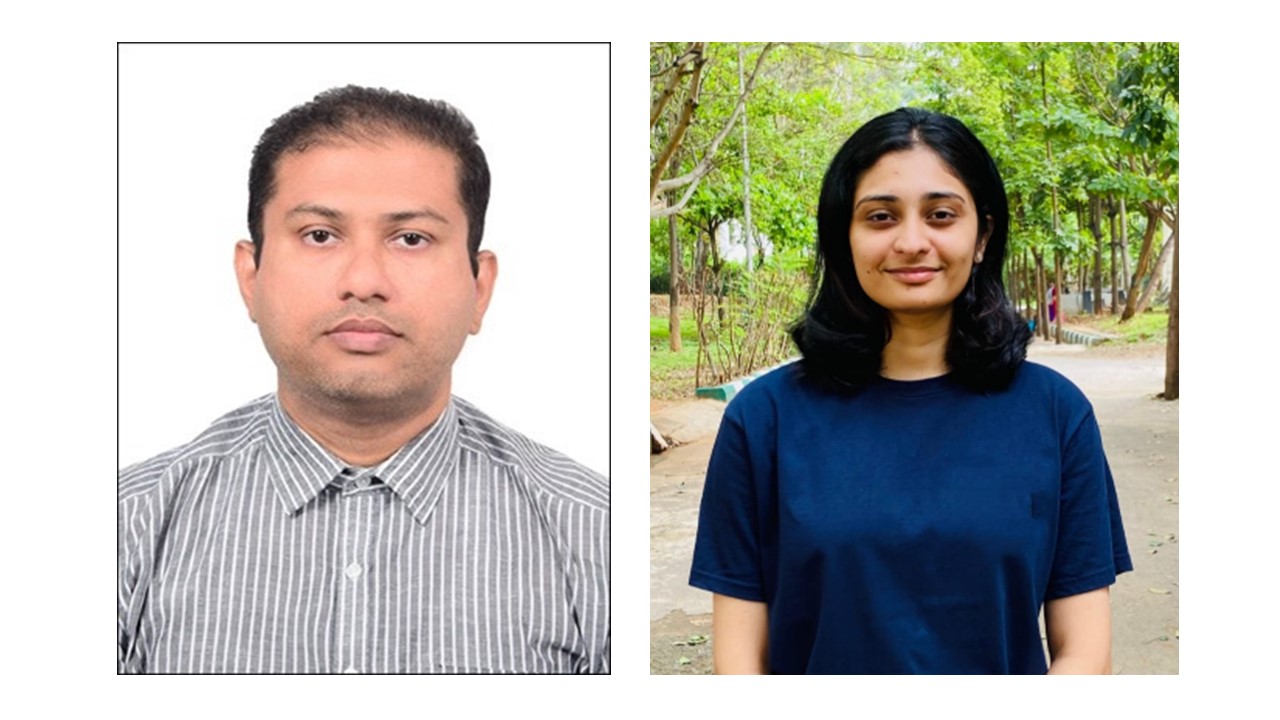
Dr. Arindam Deb, Lead Scientist (Bioinformatics) in Happiest Minds, is a profound researcher in the domain of Bioinformatics with intense research experience in academics and industry. He holds a PhD from the University of Calcutta with a national-level fellowship (RFSMS, UGC, Govt of India). He has worked in various organizations as a senior domain expert, principal scientist, and assistant professor, collaborating with national and international scientific bodies. He is also involved in teaching and mentoring as an honorary member of the Doctoral reviewer committee of renowned universities. Dr. Arindam has a keen research interest in the transformation and heterogeneity of gene regulation in various disease conditions. His present research focuses on exploring the complex disease microenvironment at single-cell resolution.
Currently, at Happiest Minds, he is advancing fundamental research in Bioinformatics in collaboration with SKAN Research Trust.
Akshatha Shetty B is an Associate Data Scientist at Happiest Minds with expertise in automating bioinformatics workflows and applying data science techniques to genomic data analysis. She holds a strong academic background in biological sciences and a master’s degree in bioinformatics. Her specialization lies in analyzing next-generation sequencing (NGS) data, with a particular focus on transcriptomics and epigenetics. Currently, she is a part of the SKAN Bioinformatics module, working on the development of a multi-omics platform.