In the realm of cybersecurity, distinguishing between real threats and false alarms is paramount. Often, alerts that initially seem critical, such as “Critical Warning: Unusual Activity Detected,” turn out to be false positives. While these instances might appear trivial, they highlight the importance of sophisticated alert management to prevent overlooking real threats, which could lead to significant security breaches.
To address this challenge and reduce false positives, the adoption of generative AI has become increasingly popular. This technology enhances the accuracy of threat detection by analysing and learning from vast datasets, allowing cybersecurity teams to focus their efforts on genuine threats.
Ignoring or improperly managing false positives has historically resulted in considerable security breaches and extensive damage. Here are a few examples:
- Colonial Pipeline Ransomware Attack (2021): In May 2021, Colonial Pipeline suffered a ransomware attack that led to widespread fuel shortages across the U.S. East Coast. Initial alerts that flagged suspicious activity were ignored due to a history of false positives in the system. The assumption that it could be another false flag resulted in a delayed response and exacerbated the impact of the attack.
- Medibank Data Breach (2022): In October 2022, Medibank, a major Australian health insurer, experienced a significant data breach affecting millions of clients. The breach investigation revealed that certain early warning signs, such as unusual access patterns and elevated privileges, were dismissed as false positives. This allowed the attackers more time within the network to exfiltrate data.
- Viasat Modem Hack (2022): In 2022, a cyberattack targeted Viasat, impacting modems and satellite communication in Ukraine and other parts of Europe. This was particularly significant due to the ongoing conflict in the region. Early logs showing anomalies in modem communication patterns were initially dismissed as technical glitches or false positives. By the time the legitimate threat was recognized, the attack had already significantly disrupted communications.
- Financial Sector Cyberattack in Asia (2024): In mid-2024, a coordinated cyberattack targeted multiple financial institutions across several Asian countries, leading to significant disruptions in banking services and financial transactions. Before the full-scale attack, there were multiple alerts regarding irregular network traffic and unauthorized access attempts. These alerts were repeatedly dismissed by the cybersecurity teams as false positives due to similar harmless incidents in the past. This led to a critical delay in detecting and mitigating the attack, causing widespread financial chaos and customer data breaches.
A common thread among these incidents is the overwhelming volume of false positives and the resultant delays in response. Burdened by a high volume of alerts, analysts often experience fatigue, leading to missed or delayed responses to genuine threats. Despite advancements in automation and streamlined processes, some alerts still slip through the cracks.
To effectively tackle these challenges, we need to embrace innovative approaches that can prevent critical alerts from being overlooked. This is where Generative AI (GenAI) can make a significant impact. By leveraging GenAI, we have the potential to revolutionize cybersecurity operations in several ways:
- Identifying Complex Attack Patterns: GenAI can spot complex attack patterns that traditional security systems might miss. It can analyze large amounts of data, recognize subtle anomalies, and provide early warnings. This strengthens an organization’s security by identifying and mitigating even the most covert threats.
- Filtering Out False Positives: One of the major challenges in cybersecurity is the high volume of false positives that analysts must deal with daily. GenAI efficiently filters out these false positives by learning from past incidents and recognizing benign activities, reducing the number of false alarms and allowing analysts to focus on real threats.
- Continuous Learning and Adaptation: The cybersecurity landscape is always changing, with new threats constantly emerging. GenAI models continuously learn from new alerts and analyst actions, allowing them to adapt to new threat patterns and improve their detection capabilities over time.
- Enhancing Incident Response: GenAI detects threats and assists in incident response. It analyses historical data and past responses to similar incidents to suggest remediation steps and recommend actions, helping analysts respond more quickly and effectively to reduce potential damage from cyberattacks.
- Automating Routine Tasks: Cybersecurity analysts can save time and reduce human error by using GenAI to automate routine tasks like monitoring network traffic, analysing logs, and generating reports.
- Predictive Analysis: GenAI performs predictive analysis by identifying trends and patterns in historical data, enabling organizations to anticipate and prevent potential future threats and enhancing security posture.
The GenAI model can be trained on vast amounts of data to recognize patterns and generate insights that can distinguish between false and real alerts. The process of training the model starts with:
- Data Preprocessing: This involves collecting telemetry data from various endpoints, such as network logs, endpoint data, threat intelligence feeds, etc. The quality and diversity of this data are crucial for creating a balanced approach to training data, leading to more accurate threat detection.
- Training the Model: GenAI models require extensive training on datasets containing examples of both real threats and false alerts, ensuring that they accurately differentiate between genuine threats and benign activities.
- Pattern Recognition: Once trained, GenAI can excel at recognizing threat alert patterns in real-time and classifying them as potential threats or benign activities, continuously learning and adapting to emerging threats.
- Reduced False Positives: The GenAI model effectively detects false alerts by understanding context and various data points, providing summarized information to help analysts filter out false alerts confidently, reducing alert fatigue.
While GenAI offers significant benefits, there are challenges to consider:
- Data Requirements: Generative AI models need massive amounts of high-quality, diverse data. Such collection is extremely difficult for organizations with limited resources or under stringent regulations. Without enough data, models run the risk of being biased or incomplete and thus perform poorly in the real world.
- Complexity and Expertise: Implementing generative AI requires knowledge of cybersecurity and machine learning. Finding or training skilled people in these two fields will be difficult and costly, making it a major challenge to deploy generative AI.
- Cost and Resource Allocation: The development and maintenance expenses of generative AI models are very high. These initiatives demand big investments in finance, infrastructure, and talent. Balancing expenses with benefits ensures the sustainability of AI initiatives.
- Integration with Existing Systems: Integrating generative AI with existing security systems presents a problematically technical task. Interaction with current tools, whether through SIEM systems or firewalls, might not be seamless and will require custom solutions, raising the difficulty level in a different direction.
Conclusion
GenAI has emerged as a powerful catalyst in our workflows and business operations, extending its impact beyond cybersecurity to every domain in today’s world. This blog introduces the basics of integrating GenAI into security operations to enhance workflow efficiency, effectiveness, and resilience against new and emerging threats. The insights provided here serve as foundational steps for organizations looking to embark on their journey with GenAI in cybersecurity.
Happiest Minds is at the forefront of developing and integrating GenAI into cybersecurity workflows. Our proprietary solution, SecAiGenie, marks a significant advancement in this direction. SecAiGenie, a GenAI-based Threat Detection and Incident Response solution, is designed to perform multiple tasks swiftly—triaging, analyzing, and providing remediation for alerts. This innovative solution significantly improves the efficiency and precision of security operations, empowering teams to concentrate on real threats with greater confidence and accuracy.
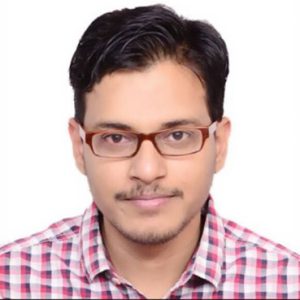
is a dedicated Associate Architect in IMSS BU with a deep passion for SOAR automation, cybersecurity, and Generative AI. With over nine years of industry experience, Mukesh excels in integrating advanced security solutions that streamline operations and enhance threat detection. He is committed to empowering teams by implementing efficient automation processes and guiding them to leverage cutting-edge technologies for real-world security challenges. Mukesh also focuses on improving security operations through the seamless integration of SOAR and Generative AI, driving innovation in cybersecurity.